Tips On How To Be A Good Student In Machine Learning
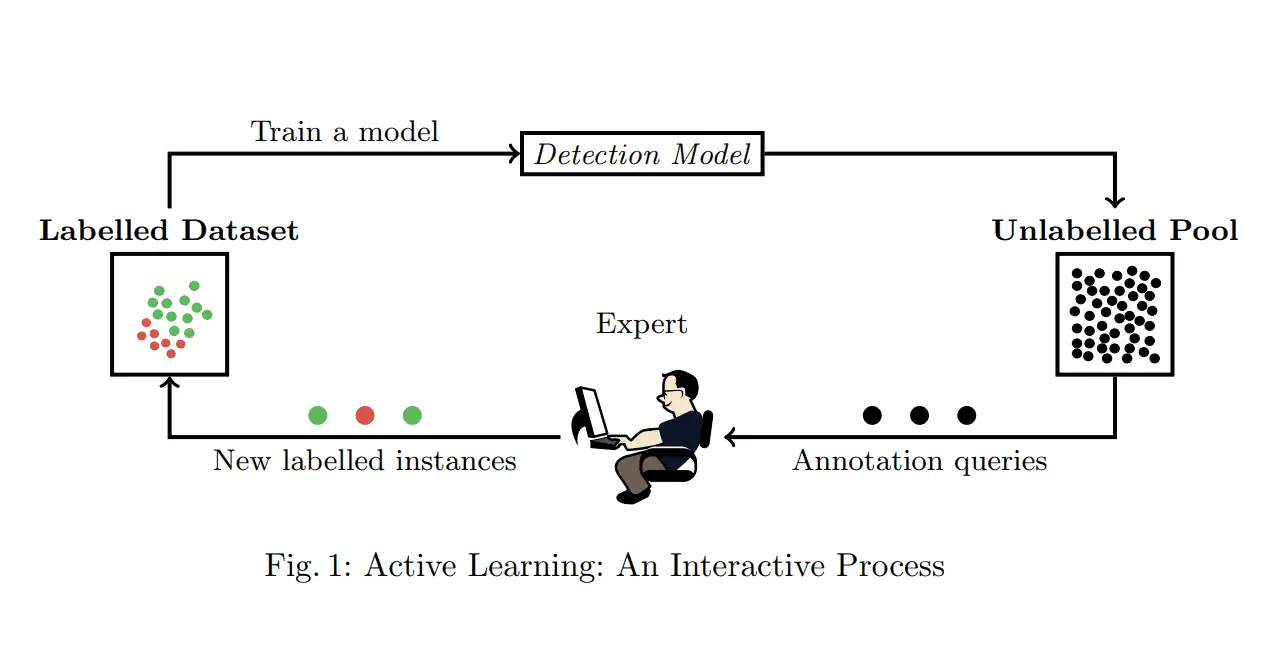
Machine learning is the process of teaching computers to learn and predict patterns from data. Usually, the aim is for the computers to get to a level to identify these patterns without prior programming. Machine learning is studied alongside similar subjects such as data analysis, artificial intelligence, and computational statistics. However, it is a large and fascinating field because students are now developing a keen interest. You can become better at machine learning in several ways, and here, you can find out how.
- Improving your expertise while learning by yourself
First, it is essential to realize that improving your machine learning is something that anyone can do. It does not matter where you are learning the skills. For instance, many students have become successful in machine learning by teaching themselves how it works. Then again, you can choose the boot camp method and learn from professionals through a boot camp. Both approaches have their advantages, and one is just as effective as the other. - What basic subjects you need to master as you begin
If you are self-taught, you need to keep in mind a couple of things. Start by teaching yourself some basic concepts within key foundational subjects. For instance, you need to practice mathematics, statistics, and also programming. Doing this will put you a step ahead of the rest. Not only will you have an easier time transitioning into more complicated concepts, but you will also build your self-confidence. With a firm grasp of mathematics and some coding, you will feel more empowered to take on machine learning algorithms and processes. Whether you have always struggled with mathematics or not, anyone can improve their skills with enough practice and achieve success. - Why machine learning theory is important
Next, you need to learn the theory behind machine learning. Most students often feel the need to jump immediately into practical understanding at this point. However, you must be a little patient. Set aside some time to explore how machine learning came about, how it has grown over the years, and how it has shaped the digital world today. Doing this will help you appreciate the subject better and understand why certain things work the way they do. Learners who are taking a new subject have always found it difficult to know how it works in practice without sufficient background knowledge on the theoretical aspect. - Practicing the basic skills
At this point, you can now begin to dive into the essential topics under machine learning and do some targeted practice within each of them. That is especially important because you are laying the groundwork for the other diverse aspects of machine learning you will encounter as you progress with your studies. With these basics, you will gain expertise and become more prepared to handle complex processes under the subject. You can start by practicing data collection, then move on to data cleaning and pre-processing. Every student of machine learning should at least know how this works. Then, you can move on to the model building, model tuning, and then evaluation. You can now start to work with real datasets and see what model works with what kind of problem. - Finding help on Homeworkdoer and other resources
Just because you are self-taught, it does not mean that you do everything on your own. As a new learner, having the right resources at your disposal will make your journey more comfortable and more enjoyable. For instance, there are multiple free machine learning programs that you can use to build on basic subjects like statistics and programming. You can find these online. When you are struggling with your homework in these subjects, you can contact the experts above. They have years of practice in their fields and will help you become good at math and programming.
Conclusion
In the end, the hours you spend practicing your skills are best used when you start the right way and master the basics before moving on to complex tasks. Enjoy this learning process, and take advantage of any professional help you can get.